Benchmarking organizations with the Dimensions API¶
This Python notebook shows how to use the Dimensions Analytics API in order to perform different benchmarking analyses of Organizations using publications data.
Outline
Quick yet effective benchmarking calculations via built-in API aggregate indicators
Building more complex quality benchmarking indicators
[2]:
import datetime
print("==\nCHANGELOG\nThis notebook was last run on %s\n==" % datetime.date.today().strftime('%b %d, %Y'))
==
CHANGELOG
This notebook was last run on Feb 21, 2022
==
Prerequisites¶
This notebook assumes you have installed the Dimcli library and are familiar with the ‘Getting Started’ tutorial.
[3]:
!pip install dimcli -U --quiet
import dimcli
from dimcli.utils import *
import os, sys, time, json
import pandas as pd
print("==\nLogging in..")
# https://digital-science.github.io/dimcli/getting-started.html#authentication
ENDPOINT = "https://app.dimensions.ai"
if 'google.colab' in sys.modules:
import getpass
KEY = getpass.getpass(prompt='API Key: ')
dimcli.login(key=KEY, endpoint=ENDPOINT)
else:
KEY = ""
dimcli.login(key=KEY, endpoint=ENDPOINT)
dsl = dimcli.Dsl()
Searching config file credentials for 'https://app.dimensions.ai' endpoint..
==
Logging in..
Dimcli - Dimensions API Client (v0.9.6)
Connected to: <https://app.dimensions.ai/api/dsl> - DSL v2.0
Method: dsl.ini file
1. Quick benchmarking using the API¶
Benchmarking by volume is reasonably straight forward if what you want to compare is volume, or one of the available aggregate indicators in the Dimensions API (see https://docs.dimensions.ai/dsl/examples.html#indicators-aggregations).
[4]:
%%dsldf
search publications
return research_orgs[name] aggregate altmetric_median
Returned Research_orgs: 20
Time: 21.14s
[4]:
altmetric_median | count | id | name | |
---|---|---|---|---|
0 | 5.0 | 546592 | grid.38142.3c | Harvard University |
1 | 3.0 | 484017 | grid.26999.3d | University of Tokyo |
2 | 4.0 | 342764 | grid.17063.33 | University of Toronto |
3 | 3.0 | 320966 | grid.214458.e | University of Michigan |
4 | 3.0 | 310485 | grid.258799.8 | Kyoto University |
5 | 4.0 | 302094 | grid.168010.e | Stanford University |
6 | 4.0 | 297558 | grid.34477.33 | University of Washington |
7 | 3.0 | 297094 | grid.19006.3e | University of California, Los Angeles |
8 | 5.0 | 289280 | grid.4991.5 | University of Oxford |
9 | 4.0 | 285143 | grid.21107.35 | Johns Hopkins University |
10 | 4.0 | 282170 | grid.5335.0 | University of Cambridge |
11 | 2.0 | 280405 | grid.11899.38 | University of São Paulo |
12 | 4.0 | 271170 | grid.25879.31 | University of Pennsylvania |
13 | 4.0 | 266337 | grid.83440.3b | University College London |
14 | 3.0 | 265592 | grid.136593.b | Osaka University |
15 | 3.0 | 250749 | grid.69566.3a | Tohoku University |
16 | 3.0 | 244713 | grid.5386.8 | Cornell University |
17 | 4.0 | 242749 | grid.47840.3f | University of California, Berkeley |
18 | 3.0 | 239283 | grid.17635.36 | University of Minnesota |
19 | 4.0 | 236142 | grid.21729.3f | Columbia University |
[5]:
%%dsldf
search publications
return research_orgs[name] aggregate citations_total
Returned Research_orgs: 20
Time: 6.63s
[5]:
citations_total | count | id | name | |
---|---|---|---|---|
0 | 28836616.0 | 546592 | grid.38142.3c | Harvard University |
1 | 8545148.0 | 484017 | grid.26999.3d | University of Tokyo |
2 | 11040840.0 | 342764 | grid.17063.33 | University of Toronto |
3 | 11710248.0 | 320966 | grid.214458.e | University of Michigan |
4 | 5928948.0 | 310485 | grid.258799.8 | Kyoto University |
5 | 14738599.0 | 302094 | grid.168010.e | Stanford University |
6 | 12585381.0 | 297558 | grid.34477.33 | University of Washington |
7 | 11710928.0 | 297094 | grid.19006.3e | University of California, Los Angeles |
8 | 10879614.0 | 289280 | grid.4991.5 | University of Oxford |
9 | 12084053.0 | 285143 | grid.21107.35 | Johns Hopkins University |
10 | 10814051.0 | 282170 | grid.5335.0 | University of Cambridge |
11 | 4105653.0 | 280405 | grid.11899.38 | University of São Paulo |
12 | 10450691.0 | 271170 | grid.25879.31 | University of Pennsylvania |
13 | 9614297.0 | 266337 | grid.83440.3b | University College London |
14 | 4653874.0 | 265592 | grid.136593.b | Osaka University |
15 | 3694359.0 | 250749 | grid.69566.3a | Tohoku University |
16 | 9370701.0 | 244713 | grid.5386.8 | Cornell University |
17 | 11806056.0 | 242749 | grid.47840.3f | University of California, Berkeley |
18 | 8360048.0 | 239283 | grid.17635.36 | University of Minnesota |
19 | 9400497.0 | 236142 | grid.21729.3f | Columbia University |
[6]:
%%dsldf
search publications
return research_orgs[name] aggregate recent_citations_total
Returned Research_orgs: 20
Time: 6.54s
[6]:
count | id | name | recent_citations_total | |
---|---|---|---|---|
0 | 546592 | grid.38142.3c | Harvard University | 5562378.0 |
1 | 484017 | grid.26999.3d | University of Tokyo | 1471000.0 |
2 | 342764 | grid.17063.33 | University of Toronto | 2380994.0 |
3 | 320966 | grid.214458.e | University of Michigan | 2370219.0 |
4 | 310485 | grid.258799.8 | Kyoto University | 1006685.0 |
5 | 302094 | grid.168010.e | Stanford University | 2985116.0 |
6 | 297558 | grid.34477.33 | University of Washington | 2411827.0 |
7 | 297094 | grid.19006.3e | University of California, Los Angeles | 2137101.0 |
8 | 289280 | grid.4991.5 | University of Oxford | 2504619.0 |
9 | 285143 | grid.21107.35 | Johns Hopkins University | 2352686.0 |
10 | 282170 | grid.5335.0 | University of Cambridge | 2110364.0 |
11 | 280405 | grid.11899.38 | University of São Paulo | 1124894.0 |
12 | 271170 | grid.25879.31 | University of Pennsylvania | 2049126.0 |
13 | 266337 | grid.83440.3b | University College London | 2197569.0 |
14 | 265592 | grid.136593.b | Osaka University | 727151.0 |
15 | 250749 | grid.69566.3a | Tohoku University | 644246.0 |
16 | 244713 | grid.5386.8 | Cornell University | 1809884.0 |
17 | 242749 | grid.47840.3f | University of California, Berkeley | 2057506.0 |
18 | 239283 | grid.17635.36 | University of Minnesota | 1519539.0 |
19 | 236142 | grid.21729.3f | Columbia University | 1754780.0 |
Aside: Recent Citations
[7]:
%%dsldf
search publications
return year aggregate recent_citations_total
Returned Year: 20
Time: 4.06s
[7]:
count | id | recent_citations_total | |
---|---|---|---|
0 | 6503486 | 2020 | 18375337.0 |
1 | 6391947 | 2021 | 4632716.0 |
2 | 5792555 | 2019 | 22470145.0 |
3 | 5369555 | 2018 | 23030935.0 |
4 | 5044596 | 2017 | 21362603.0 |
5 | 4598245 | 2016 | 19046830.0 |
6 | 4395107 | 2015 | 17010283.0 |
7 | 4244049 | 2014 | 15057104.0 |
8 | 4046162 | 2013 | 13475978.0 |
9 | 3762532 | 2012 | 11970228.0 |
10 | 3667073 | 2011 | 10958039.0 |
11 | 3430544 | 2010 | 9915351.0 |
12 | 3144460 | 2009 | 8991871.0 |
13 | 2937393 | 2008 | 7853718.0 |
14 | 2915691 | 2007 | 7198101.0 |
15 | 2610760 | 2006 | 6579372.0 |
16 | 2410569 | 2005 | 5985630.0 |
17 | 2246194 | 2004 | 5335870.0 |
18 | 2037978 | 2003 | 4730168.0 |
19 | 1892417 | 2002 | 4234096.0 |
[8]:
dsl_last_results.sort_values(by='id').plot(x='id', y='recent_citations_total', figsize=(20,10))
[8]:
<AxesSubplot:xlabel='id'>
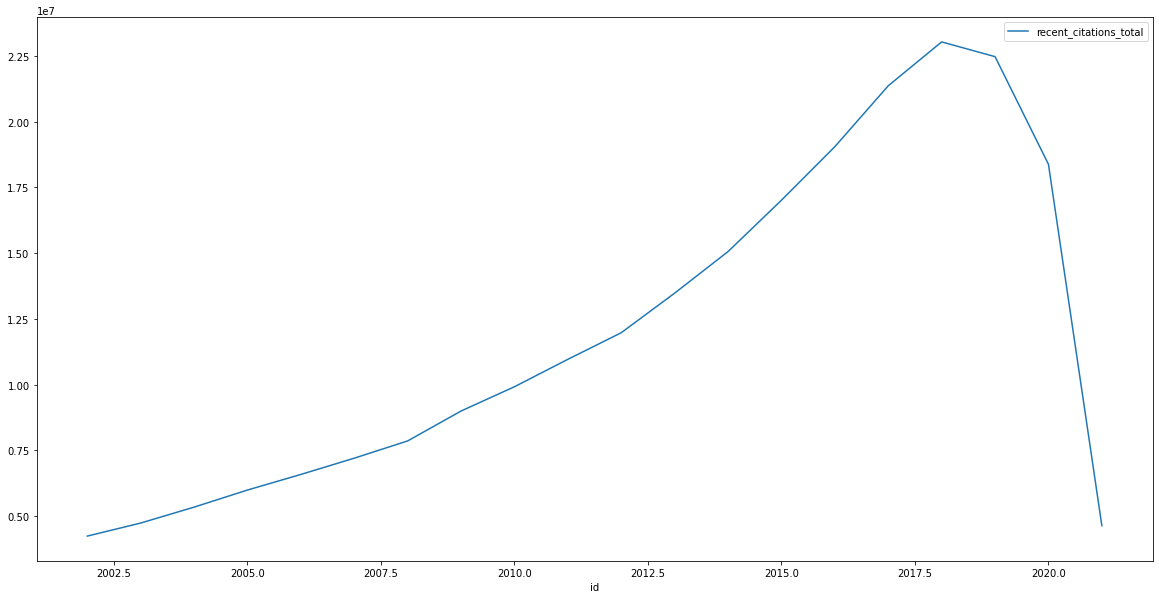
[9]:
recent_citations = dsl_last_results
[10]:
recent_citations['recent_ratio'] = recent_citations['recent_citations_total']/recent_citations['count']
recent_citations['year'] = recent_citations['id']
[11]:
recent_citations.sort_values(by='year').\
plot(x='year',y='recent_ratio', figsize=(20,10))
[11]:
<AxesSubplot:xlabel='year'>
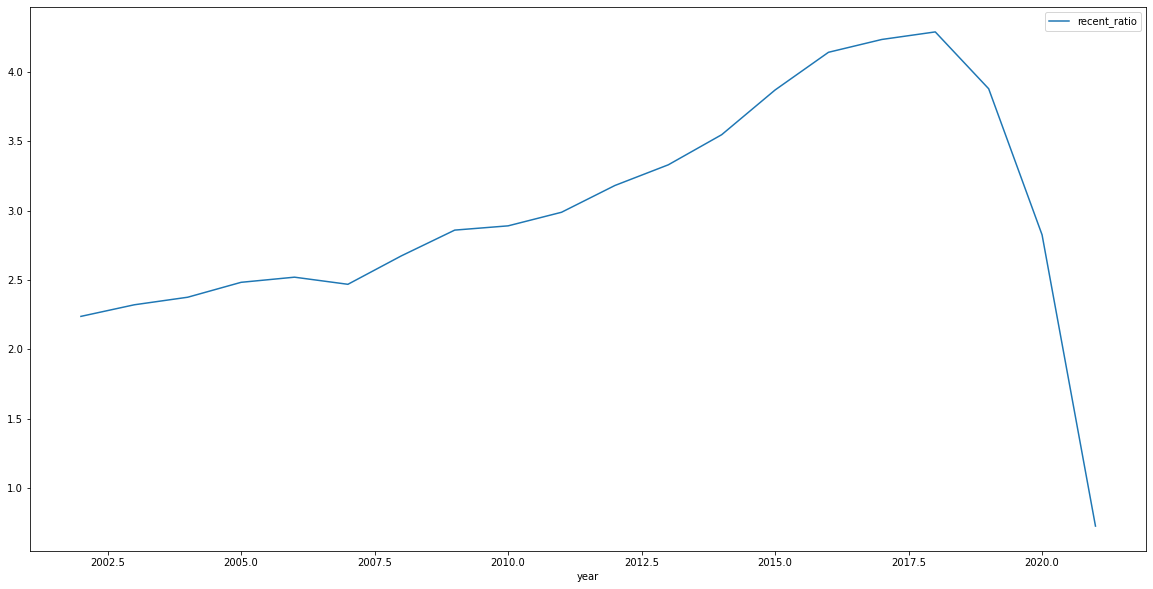
End Aside:
2. Calculating more complex ‘Quality’ Benchmarking indicators: Number of articles in the top X percent of research their category¶
Step 1. retrieve the total volume of publications by volume. (focusing on Fields of Research)¶
[12]:
%%dsldf
search publications
where year=2018
return category_for limit 1000
Returned Category_for: 176
Time: 1.02s
[12]:
count | id | name | |
---|---|---|---|
0 | 1168442 | 2211 | 11 Medical and Health Sciences |
1 | 610238 | 2209 | 09 Engineering |
2 | 447354 | 3053 | 1103 Clinical Sciences |
3 | 335403 | 2206 | 06 Biological Sciences |
4 | 332128 | 2208 | 08 Information and Computing Sciences |
... | ... | ... | ... |
171 | 187 | 3528 | 1899 Other Law and Legal Studies |
172 | 144 | 3491 | 1799 Other Psychology and Cognitive Sciences |
173 | 72 | 3567 | 1999 Other Studies In Creative Arts and Writing |
174 | 62 | 3240 | 1299 Other Built Environment and Design |
175 | 21 | 3223 | 1204 Engineering Design |
176 rows × 3 columns
Step 1.2. … Need to filter for level 2 codes¶
[13]:
result = dsl.query("""
search publications
where year=2018
return category_for limit 1000
""").as_dataframe()
Returned Category_for: 176
Time: 0.84s
[14]:
result['level'] = result.name.apply(lambda n: len(n.split(' ')[0]))
[15]:
result
[15]:
count | id | name | level | |
---|---|---|---|---|
0 | 1168442 | 2211 | 11 Medical and Health Sciences | 2 |
1 | 610238 | 2209 | 09 Engineering | 2 |
2 | 447354 | 3053 | 1103 Clinical Sciences | 4 |
3 | 335403 | 2206 | 06 Biological Sciences | 2 |
4 | 332128 | 2208 | 08 Information and Computing Sciences | 2 |
... | ... | ... | ... | ... |
171 | 187 | 3528 | 1899 Other Law and Legal Studies | 4 |
172 | 144 | 3491 | 1799 Other Psychology and Cognitive Sciences | 4 |
173 | 72 | 3567 | 1999 Other Studies In Creative Arts and Writing | 4 |
174 | 62 | 3240 | 1299 Other Built Environment and Design | 4 |
175 | 21 | 3223 | 1204 Engineering Design | 4 |
176 rows × 4 columns
[16]:
result[result['level']==2]
[16]:
count | id | name | level | |
---|---|---|---|---|
0 | 1168442 | 2211 | 11 Medical and Health Sciences | 2 |
1 | 610238 | 2209 | 09 Engineering | 2 |
3 | 335403 | 2206 | 06 Biological Sciences | 2 |
4 | 332128 | 2208 | 08 Information and Computing Sciences | 2 |
5 | 304680 | 2203 | 03 Chemical Sciences | 2 |
7 | 224973 | 2202 | 02 Physical Sciences | 2 |
8 | 201573 | 2201 | 01 Mathematical Sciences | 2 |
12 | 161476 | 2217 | 17 Psychology and Cognitive Sciences | 2 |
13 | 151455 | 2216 | 16 Studies in Human Society | 2 |
18 | 98630 | 2215 | 15 Commerce, Management, Tourism and Services | 2 |
20 | 95061 | 2210 | 10 Technology | 2 |
21 | 94318 | 2220 | 20 Language, Communication and Culture | 2 |
24 | 88929 | 2213 | 13 Education | 2 |
25 | 86868 | 2204 | 04 Earth Sciences | 2 |
26 | 85471 | 2214 | 14 Economics | 2 |
27 | 80461 | 2221 | 21 History and Archaeology | 2 |
32 | 71522 | 2205 | 05 Environmental Sciences | 2 |
35 | 67805 | 2207 | 07 Agricultural and Veterinary Sciences | 2 |
41 | 56606 | 2222 | 22 Philosophy and Religious Studies | 2 |
48 | 43353 | 2218 | 18 Law and Legal Studies | 2 |
74 | 26972 | 2212 | 12 Built Environment and Design | 2 |
84 | 20301 | 2219 | 19 Studies in Creative Arts and Writing | 2 |
Step 2. calculate 1% of the total number of records by category. This will be used to retrieve the 1% boundary record..¶
What is the boundary record?
[17]:
result['cutoff'] = (result['count'] * .01).astype('int')
[18]:
result[result['level']==2]
[18]:
count | id | name | level | cutoff | |
---|---|---|---|---|---|
0 | 1168442 | 2211 | 11 Medical and Health Sciences | 2 | 11684 |
1 | 610238 | 2209 | 09 Engineering | 2 | 6102 |
3 | 335403 | 2206 | 06 Biological Sciences | 2 | 3354 |
4 | 332128 | 2208 | 08 Information and Computing Sciences | 2 | 3321 |
5 | 304680 | 2203 | 03 Chemical Sciences | 2 | 3046 |
7 | 224973 | 2202 | 02 Physical Sciences | 2 | 2249 |
8 | 201573 | 2201 | 01 Mathematical Sciences | 2 | 2015 |
12 | 161476 | 2217 | 17 Psychology and Cognitive Sciences | 2 | 1614 |
13 | 151455 | 2216 | 16 Studies in Human Society | 2 | 1514 |
18 | 98630 | 2215 | 15 Commerce, Management, Tourism and Services | 2 | 986 |
20 | 95061 | 2210 | 10 Technology | 2 | 950 |
21 | 94318 | 2220 | 20 Language, Communication and Culture | 2 | 943 |
24 | 88929 | 2213 | 13 Education | 2 | 889 |
25 | 86868 | 2204 | 04 Earth Sciences | 2 | 868 |
26 | 85471 | 2214 | 14 Economics | 2 | 854 |
27 | 80461 | 2221 | 21 History and Archaeology | 2 | 804 |
32 | 71522 | 2205 | 05 Environmental Sciences | 2 | 715 |
35 | 67805 | 2207 | 07 Agricultural and Veterinary Sciences | 2 | 678 |
41 | 56606 | 2222 | 22 Philosophy and Religious Studies | 2 | 566 |
48 | 43353 | 2218 | 18 Law and Legal Studies | 2 | 433 |
74 | 26972 | 2212 | 12 Built Environment and Design | 2 | 269 |
84 | 20301 | 2219 | 19 Studies in Creative Arts and Writing | 2 | 203 |
Step 3. Use the cutoff value to get the indicator value for the 1% boundary¶
Note: Here we use:
‘sort by’ , limit, and skip!
‘sort by’: return results in order of field_citation_ratio
‘limit’: we are only interested in the first result returned
‘skip’ we are ‘skipping’ to the boundary record
Double Note: this strategy won’t work when the boundary record is > 50,000…
[19]:
dfl = []
for r in result[result['level']==2].iterrows():
result = dsl.query(f"""
search publications
where category_for.id = "{r[1]['id']}"
and year = 2018
return publications[field_citation_ratio]
sort by field_citation_ratio
limit 1
skip {r[1]['cutoff']}
""").as_dataframe()
result['name'] = r[1]['name']
result['id'] = r[1]['id']
dfl.append(result)
Returned Publications: 1 (total = 1168442)
Time: 9.13s
Returned Publications: 1 (total = 610238)
Time: 4.71s
Returned Publications: 1 (total = 335403)
Time: 2.55s
Returned Publications: 1 (total = 332128)
Time: 2.55s
Returned Publications: 1 (total = 304680)
Time: 2.17s
Returned Publications: 1 (total = 224973)
Time: 2.28s
Returned Publications: 1 (total = 201573)
Time: 2.07s
Returned Publications: 1 (total = 161476)
Time: 1.58s
Returned Publications: 1 (total = 151455)
Time: 1.67s
Returned Publications: 1 (total = 98630)
Time: 1.27s
Returned Publications: 1 (total = 95061)
Time: 0.91s
Returned Publications: 1 (total = 94318)
Time: 1.18s
Returned Publications: 1 (total = 88929)
Time: 1.07s
Returned Publications: 1 (total = 86868)
Time: 1.03s
Returned Publications: 1 (total = 85471)
Time: 1.12s
Returned Publications: 1 (total = 80461)
Time: 1.27s
Returned Publications: 1 (total = 71522)
Time: 0.92s
Returned Publications: 1 (total = 67805)
Time: 1.14s
Returned Publications: 1 (total = 56606)
Time: 1.06s
Returned Publications: 1 (total = 43353)
Time: 0.86s
Returned Publications: 1 (total = 26972)
Time: 0.75s
Returned Publications: 1 (total = 20301)
Time: 0.82s
[20]:
cutoffs = pd.concat(dfl)
[21]:
cutoffs
[21]:
field_citation_ratio | name | id | |
---|---|---|---|
0 | 28.41 | 11 Medical and Health Sciences | 2211 |
0 | 21.35 | 09 Engineering | 2209 |
0 | 20.52 | 06 Biological Sciences | 2206 |
0 | 35.44 | 08 Information and Computing Sciences | 2208 |
0 | 20.51 | 03 Chemical Sciences | 2203 |
0 | 24.72 | 02 Physical Sciences | 2202 |
0 | 27.12 | 01 Mathematical Sciences | 2201 |
0 | 24.56 | 17 Psychology and Cognitive Sciences | 2217 |
0 | 27.91 | 16 Studies in Human Society | 2216 |
0 | 32.01 | 15 Commerce, Management, Tourism and Services | 2215 |
0 | 25.02 | 10 Technology | 2210 |
0 | 30.45 | 20 Language, Communication and Culture | 2220 |
0 | 25.34 | 13 Education | 2213 |
0 | 16.52 | 04 Earth Sciences | 2204 |
0 | 33.18 | 14 Economics | 2214 |
0 | 28.80 | 21 History and Archaeology | 2221 |
0 | 20.46 | 05 Environmental Sciences | 2205 |
0 | 15.42 | 07 Agricultural and Veterinary Sciences | 2207 |
0 | 27.68 | 22 Philosophy and Religious Studies | 2222 |
0 | 27.52 | 18 Law and Legal Studies | 2218 |
0 | 16.68 | 12 Built Environment and Design | 2212 |
0 | 27.55 | 19 Studies in Creative Arts and Writing | 2219 |
We can only filter on integers in the DSL, so we will round up the values¶
[22]:
cutoffs.field_citation_ratio = cutoffs.field_citation_ratio.astype('int')
[23]:
cutoffs
[23]:
field_citation_ratio | name | id | |
---|---|---|---|
0 | 28 | 11 Medical and Health Sciences | 2211 |
0 | 21 | 09 Engineering | 2209 |
0 | 20 | 06 Biological Sciences | 2206 |
0 | 35 | 08 Information and Computing Sciences | 2208 |
0 | 20 | 03 Chemical Sciences | 2203 |
0 | 24 | 02 Physical Sciences | 2202 |
0 | 27 | 01 Mathematical Sciences | 2201 |
0 | 24 | 17 Psychology and Cognitive Sciences | 2217 |
0 | 27 | 16 Studies in Human Society | 2216 |
0 | 32 | 15 Commerce, Management, Tourism and Services | 2215 |
0 | 25 | 10 Technology | 2210 |
0 | 30 | 20 Language, Communication and Culture | 2220 |
0 | 25 | 13 Education | 2213 |
0 | 16 | 04 Earth Sciences | 2204 |
0 | 33 | 14 Economics | 2214 |
0 | 28 | 21 History and Archaeology | 2221 |
0 | 20 | 05 Environmental Sciences | 2205 |
0 | 15 | 07 Agricultural and Veterinary Sciences | 2207 |
0 | 27 | 22 Philosophy and Religious Studies | 2222 |
0 | 27 | 18 Law and Legal Studies | 2218 |
0 | 16 | 12 Built Environment and Design | 2212 |
0 | 27 | 19 Studies in Creative Arts and Writing | 2219 |
Step 4. Now get the number of publications by organisation, filtered by category that have a field_citation_ratio > the boundary score¶
[24]:
dfl = []
for r in cutoffs.iterrows():
result = dsl.query(f"""
search publications
where
year=2018
and category_for.id = "{r[1]['id']}"
and field_citation_ratio >= {int(r[1]['field_citation_ratio'])}
return research_orgs limit 1000
""").as_dataframe()
result['for_name'] = r[1]['name']
result['for_id'] = r[1]['id']
dfl.append(result)
Returned Research_orgs: 1000
Time: 1.21s
Returned Research_orgs: 1000
Time: 1.09s
Returned Research_orgs: 1000
Time: 3.14s
Returned Research_orgs: 1000
Time: 1.30s
Returned Research_orgs: 1000
Time: 0.96s
Returned Research_orgs: 1000
Time: 1.13s
Returned Research_orgs: 1000
Time: 1.06s
Returned Research_orgs: 1000
Time: 1.16s
Returned Research_orgs: 1000
Time: 1.06s
Returned Research_orgs: 927
Time: 1.13s
Returned Research_orgs: 915
Time: 1.04s
Returned Research_orgs: 704
Time: 0.83s
Returned Research_orgs: 863
Time: 1.03s
Returned Research_orgs: 1000
Time: 1.01s
Returned Research_orgs: 903
Time: 0.96s
Returned Research_orgs: 896
Time: 1.10s
Returned Research_orgs: 1000
Time: 1.26s
Returned Research_orgs: 1000
Time: 1.07s
Returned Research_orgs: 476
Time: 0.81s
Returned Research_orgs: 495
Time: 0.71s
Returned Research_orgs: 369
Time: 0.75s
Returned Research_orgs: 210
Time: 0.77s
ok, can only filter on Integrers
[25]:
top_insts = pd.concat(dfl)
Step 5. Rank the results¶
[26]:
top_insts['rank'] = top_insts.groupby('for_name')['count'].rank(ascending=False)
[27]:
top_insts[top_insts['name']=='University of Melbourne'][['for_name','rank']]
[27]:
for_name | rank | |
---|---|---|
21 | 11 Medical and Health Sciences | 22.0 |
103 | 09 Engineering | 107.0 |
25 | 06 Biological Sciences | 26.0 |
99 | 08 Information and Computing Sciences | 105.0 |
161 | 03 Chemical Sciences | 170.5 |
142 | 02 Physical Sciences | 150.0 |
45 | 01 Mathematical Sciences | 48.5 |
9 | 17 Psychology and Cognitive Sciences | 11.5 |
32 | 16 Studies in Human Society | 36.0 |
66 | 15 Commerce, Management, Tourism and Services | 88.0 |
35 | 20 Language, Communication and Culture | 46.0 |
17 | 13 Education | 22.5 |
196 | 04 Earth Sciences | 230.0 |
83 | 14 Economics | 110.0 |
263 | 21 History and Archaeology | 579.5 |
30 | 05 Environmental Sciences | 34.5 |
22 | 07 Agricultural and Veterinary Sciences | 26.0 |
133 | 22 Philosophy and Religious Studies | 304.0 |
23 | 18 Law and Legal Studies | 37.0 |
20 | 12 Built Environment and Design | 32.5 |
0 | 19 Studies in Creative Arts and Writing | 1.0 |
We should probably control for Volume though…¶
Step 6. Get the total paper counts for each organisation¶
[28]:
dfl = []
for r in cutoffs.iterrows():
result = dsl.query(f"""
search publications
where
year=2018
and category_for.id = "{r[1]['id']}"
return research_orgs limit 1000
""").as_dataframe()
result['for_name'] = r[1]['name']
result['for_id'] = r[1]['id']
dfl.append(result)
Returned Research_orgs: 1000
Time: 1.47s
Returned Research_orgs: 1000
Time: 1.12s
Returned Research_orgs: 1000
Time: 1.14s
Returned Research_orgs: 1000
Time: 1.09s
Returned Research_orgs: 1000
Time: 0.97s
Returned Research_orgs: 1000
Time: 1.17s
Returned Research_orgs: 1000
Time: 1.29s
Returned Research_orgs: 1000
Time: 1.11s
Returned Research_orgs: 1000
Time: 1.00s
Returned Research_orgs: 1000
Time: 1.02s
Returned Research_orgs: 1000
Time: 0.98s
Returned Research_orgs: 1000
Time: 0.98s
Returned Research_orgs: 1000
Time: 1.03s
Returned Research_orgs: 1000
Time: 0.98s
Returned Research_orgs: 1000
Time: 0.98s
Returned Research_orgs: 1000
Time: 1.12s
Returned Research_orgs: 1000
Time: 1.14s
Returned Research_orgs: 1000
Time: 1.15s
Returned Research_orgs: 1000
Time: 1.15s
Returned Research_orgs: 1000
Time: 1.10s
Returned Research_orgs: 1000
Time: 0.97s
Returned Research_orgs: 1000
Time: 0.96s
[29]:
all_publications = pd.concat(dfl)[['id','for_id','count']]
[30]:
top_insts_all = all_publications.rename(columns={'count':'count all'}).merge(top_insts, on =['id','for_id'])
[31]:
top_insts_all[['for_name','name','count','count all']]
[31]:
for_name | name | count | count all | |
---|---|---|---|---|
0 | 11 Medical and Health Sciences | Harvard University | 845 | 16932 |
1 | 11 Medical and Health Sciences | University of Toronto | 392 | 10281 |
2 | 11 Medical and Health Sciences | Johns Hopkins University | 391 | 10120 |
3 | 11 Medical and Health Sciences | University of California, San Francisco | 365 | 7850 |
4 | 11 Medical and Health Sciences | Mayo Clinic | 321 | 7659 |
... | ... | ... | ... | ... |
12220 | 19 Studies in Creative Arts and Writing | University of Bamberg | 1 | 3 |
12221 | 19 Studies in Creative Arts and Writing | National University of Quilmes | 1 | 2 |
12222 | 19 Studies in Creative Arts and Writing | Czech University of Life Sciences Prague | 1 | 2 |
12223 | 19 Studies in Creative Arts and Writing | University Hospitals of Cleveland | 1 | 2 |
12224 | 19 Studies in Creative Arts and Writing | Grinnell College | 1 | 2 |
12225 rows × 4 columns
Step 7. calculate the percentage of local papers in the top 1% of global publications (in 2018)¶
[32]:
top_insts_all['percentage top 1'] = (100 * top_insts_all['count']/top_insts_all['count all']).round(2)
[33]:
top_insts_all['percent rank'] = top_insts_all.groupby('for_name')['percentage top 1'].rank(ascending=False)
Now the results are going to look a little strange…¶
[34]:
top_insts_all[top_insts_all['name']=='University of Cambridge'][['for_name','percent rank']]
[34]:
for_name | percent rank | |
---|---|---|
66 | 11 Medical and Health Sciences | 41.0 |
840 | 09 Engineering | 138.0 |
1498 | 06 Biological Sciences | 100.0 |
2294 | 08 Information and Computing Sciences | 475.5 |
2875 | 03 Chemical Sciences | 93.5 |
3512 | 02 Physical Sciences | 278.5 |
4277 | 01 Mathematical Sciences | 117.0 |
4921 | 17 Psychology and Cognitive Sciences | 52.5 |
5584 | 16 Studies in Human Society | 236.5 |
6165 | 15 Commerce, Management, Tourism and Services | 150.0 |
6864 | 10 Technology | 304.0 |
7312 | 20 Language, Communication and Culture | 398.5 |
7785 | 13 Education | 377.0 |
8302 | 04 Earth Sciences | 346.5 |
8940 | 14 Economics | 310.5 |
9467 | 21 History and Archaeology | 305.0 |
10013 | 05 Environmental Sciences | 123.0 |
10741 | 07 Agricultural and Veterinary Sciences | 124.5 |
11176 | 22 Philosophy and Religious Studies | 311.5 |
11503 | 18 Law and Legal Studies | 196.0 |
11823 | 12 Built Environment and Design | 181.0 |
[35]:
top_insts_all[top_insts_all['for_name']=='11 Medical and Health Sciences'][['name','percent rank']]
[35]:
name | percent rank | |
---|---|---|
0 | Harvard University | 61.5 |
1 | University of Toronto | 236.5 |
2 | Johns Hopkins University | 220.0 |
3 | University of California, San Francisco | 93.5 |
4 | Mayo Clinic | 152.0 |
... | ... | ... |
780 | University of Bath | 425.0 |
781 | Kuopio University Hospital | 250.5 |
782 | Marqués de Valdecilla University Hospital | 299.0 |
783 | Policlinico San Matteo Fondazione | 114.5 |
784 | Centre Hospitalier Universitaire de Caen | 351.5 |
785 rows × 2 columns
Smaller institutions are being preferenced too much…
Need to control for size…¶
[36]:
reference_institutions = top_insts_all[['id','name','for_id','count all']].\
rename(columns={
'id':'reference id',
'name':'reference name',
'count all':'reference count all'
})
[37]:
relative_ranking = reference_institutions.merge(top_insts_all, on='for_id')
[38]:
relative_ranking[relative_ranking['reference name']=='University of Melbourne']
[38]:
reference id | reference name | for_id | reference count all | id | count all | city_name | count | country_name | latitude | linkout | longitude | name | state_name | types | acronym | for_name | rank | percentage top 1 | percent rank | |
---|---|---|---|---|---|---|---|---|---|---|---|---|---|---|---|---|---|---|---|---|
15700 | grid.1008.9 | University of Melbourne | 2211 | 5457 | grid.38142.3c | 16932 | Cambridge | 845 | United States | 42.377052 | [http://www.harvard.edu/] | -71.116650 | Harvard University | Massachusetts | [Education] | NaN | 11 Medical and Health Sciences | 1.0 | 4.99 | 61.5 |
15701 | grid.1008.9 | University of Melbourne | 2211 | 5457 | grid.17063.33 | 10281 | Toronto | 392 | Canada | 43.661667 | [http://www.utoronto.ca/] | -79.395000 | University of Toronto | Ontario | [Education] | NaN | 11 Medical and Health Sciences | 2.0 | 3.81 | 236.5 |
15702 | grid.1008.9 | University of Melbourne | 2211 | 5457 | grid.21107.35 | 10120 | Baltimore | 391 | United States | 39.328888 | [https://www.jhu.edu/] | -76.620280 | Johns Hopkins University | Maryland | [Education] | JHU | 11 Medical and Health Sciences | 3.0 | 3.86 | 220.0 |
15703 | grid.1008.9 | University of Melbourne | 2211 | 5457 | grid.266102.1 | 7850 | San Francisco | 365 | United States | 37.762800 | [https://www.ucsf.edu/] | -122.457670 | University of California, San Francisco | California | [Education] | UCSF | 11 Medical and Health Sciences | 6.0 | 4.65 | 93.5 |
15704 | grid.1008.9 | University of Melbourne | 2211 | 5457 | grid.66875.3a | 7659 | Rochester | 321 | United States | 44.024070 | [http://www.mayoclinic.org/patient-visitor-gui... | -92.466310 | Mayo Clinic | Minnesota | [Healthcare] | NaN | 11 Medical and Health Sciences | 10.0 | 4.19 | 152.0 |
... | ... | ... | ... | ... | ... | ... | ... | ... | ... | ... | ... | ... | ... | ... | ... | ... | ... | ... | ... | ... |
7350128 | grid.1008.9 | University of Melbourne | 2219 | 85 | grid.7359.8 | 3 | Bamberg | 1 | Germany | 49.893845 | [https://www.uni-bamberg.de/] | 10.886044 | University of Bamberg | NaN | [Education] | NaN | 19 Studies in Creative Arts and Writing | 130.0 | 33.33 | 8.0 |
7350129 | grid.1008.9 | University of Melbourne | 2219 | 85 | grid.11560.33 | 2 | Bernal | 1 | Argentina | -34.706670 | [http://www.unq.edu.ar/english/sections/158-unq/] | -58.277500 | National University of Quilmes | NaN | [Education] | UNQ | 19 Studies in Creative Arts and Writing | 130.0 | 50.00 | 2.5 |
7350130 | grid.1008.9 | University of Melbourne | 2219 | 85 | grid.15866.3c | 2 | Prague | 1 | Czechia | 50.131460 | [http://www.czu.cz/en/] | 14.373258 | Czech University of Life Sciences Prague | NaN | [Education] | CULS | 19 Studies in Creative Arts and Writing | 130.0 | 50.00 | 2.5 |
7350131 | grid.1008.9 | University of Melbourne | 2219 | 85 | grid.241104.2 | 2 | Cleveland | 1 | United States | 41.506096 | [http://www.uhhospitals.org/] | -81.604820 | University Hospitals of Cleveland | Ohio | [Healthcare] | NaN | 19 Studies in Creative Arts and Writing | 130.0 | 50.00 | 2.5 |
7350132 | grid.1008.9 | University of Melbourne | 2219 | 85 | grid.256592.f | 2 | Grinnell | 1 | United States | 41.749737 | [http://www.grinnell.edu/] | -92.719505 | Grinnell College | Iowa | [Education] | NaN | 19 Studies in Creative Arts and Writing | 130.0 | 50.00 | 2.5 |
11685 rows × 20 columns
[39]:
filtered_relative_ranking = relative_ranking[relative_ranking[
'reference count all'] <= relative_ranking['count all']
].copy()
[40]:
filtered_relative_ranking['filtered percent rank'] = filtered_relative_ranking.\
groupby(['reference id','for_name'])['percentage top 1'].\
rank(ascending=False)
[41]:
inst = 'University of Melbourne'
filtered_relative_ranking[
(filtered_relative_ranking['reference name'] == inst) &
(filtered_relative_ranking['name'] == inst)
][['id', 'for_id', 'name','for_name','filtered percent rank']]
[41]:
id | for_id | name | for_name | filtered percent rank | |
---|---|---|---|---|---|
15720 | grid.1008.9 | 2211 | University of Melbourne | 11 Medical and Health Sciences | 15.5 |
738709 | grid.1008.9 | 2209 | University of Melbourne | 09 Engineering | 40.0 |
1131875 | grid.1008.9 | 2206 | University of Melbourne | 06 Biological Sciences | 13.0 |
1643602 | grid.1008.9 | 2208 | University of Melbourne | 08 Information and Computing Sciences | 45.0 |
2140491 | grid.1008.9 | 2203 | University of Melbourne | 03 Chemical Sciences | 87.5 |
2627450 | grid.1008.9 | 2202 | University of Melbourne | 02 Physical Sciences | 49.0 |
3094798 | grid.1008.9 | 2201 | University of Melbourne | 01 Mathematical Sciences | 18.0 |
3454060 | grid.1008.9 | 2217 | University of Melbourne | 17 Psychology and Cognitive Sciences | 4.0 |
3909944 | grid.1008.9 | 2216 | University of Melbourne | 16 Studies in Human Society | 4.0 |
4225053 | grid.1008.9 | 2215 | University of Melbourne | 15 Commerce, Management, Tourism and Services | 9.0 |
4915245 | grid.1008.9 | 2220 | University of Melbourne | 20 Language, Communication and Culture | 3.0 |
5111347 | grid.1008.9 | 2213 | University of Melbourne | 13 Education | 8.0 |
5429203 | grid.1008.9 | 2204 | University of Melbourne | 04 Earth Sciences | 64.0 |
5817193 | grid.1008.9 | 2214 | University of Melbourne | 14 Economics | 13.0 |
6111107 | grid.1008.9 | 2221 | University of Melbourne | 21 History and Archaeology | 22.0 |
6352914 | grid.1008.9 | 2205 | University of Melbourne | 05 Environmental Sciences | 6.0 |
6797399 | grid.1008.9 | 2207 | University of Melbourne | 07 Agricultural and Veterinary Sciences | 9.0 |
7091867 | grid.1008.9 | 2222 | University of Melbourne | 22 Philosophy and Religious Studies | 13.0 |
7194418 | grid.1008.9 | 2218 | University of Melbourne | 18 Law and Legal Studies | 4.0 |
7281134 | grid.1008.9 | 2212 | University of Melbourne | 12 Built Environment and Design | 7.0 |
7349969 | grid.1008.9 | 2219 | University of Melbourne | 19 Studies in Creative Arts and Writing | 1.0 |
[ ]:
Final step. Show me the institutions that I should be most interested in (Five above)¶
[42]:
rank_cutoffs = filtered_relative_ranking[
(filtered_relative_ranking['reference name'] == filtered_relative_ranking['name'] )
][['id', 'for_id', 'filtered percent rank']].\
rename(columns={'id':'reference id',
'filtered percent rank':'reference filtered percent rank'})
[43]:
filtered_relative_ranking_final = rank_cutoffs.merge(filtered_relative_ranking, on=['reference id','for_id'])
[44]:
filtered_relative_ranking_final['rank_difference'] = filtered_relative_ranking_final['filtered percent rank'] - filtered_relative_ranking_final['reference filtered percent rank']
[45]:
inst = 'Monash University'
forname = '11 Medical and Health Sciences'
filtered_relative_ranking_final[
(filtered_relative_ranking_final['rank_difference'].between(-5, 5)) &
(filtered_relative_ranking_final['reference name'] == inst) &
(filtered_relative_ranking_final['for_name'] == forname)
][['name','filtered percent rank']].sort_values(by='filtered percent rank')
[45]:
name | filtered percent rank | |
---|---|---|
515 | University of Michigan | 24.5 |
524 | Karolinska Institute | 24.5 |
523 | Emory University | 26.0 |
528 | University of Pittsburgh | 27.0 |
521 | University of Sydney | 28.0 |
538 | Monash University | 29.0 |
533 | University of British Columbia | 30.0 |
520 | University of São Paulo | 31.0 |
534 | Shanghai Jiao Tong University | 32.0 |
[46]:
filtered_relative_ranking_final
[46]:
reference id | for_id | reference filtered percent rank | reference name | reference count all | id | count all | city_name | count | country_name | ... | name | state_name | types | acronym | for_name | rank | percentage top 1 | percent rank | filtered percent rank | rank_difference | |
---|---|---|---|---|---|---|---|---|---|---|---|---|---|---|---|---|---|---|---|---|---|
0 | grid.38142.3c | 2211 | 1.0 | Harvard University | 16932 | grid.38142.3c | 16932 | Cambridge | 845 | United States | ... | Harvard University | Massachusetts | [Education] | NaN | 11 Medical and Health Sciences | 1.0 | 4.99 | 61.5 | 1.0 | 0.0 |
1 | grid.17063.33 | 2211 | 2.0 | University of Toronto | 10281 | grid.38142.3c | 16932 | Cambridge | 845 | United States | ... | Harvard University | Massachusetts | [Education] | NaN | 11 Medical and Health Sciences | 1.0 | 4.99 | 61.5 | 1.0 | -1.0 |
2 | grid.17063.33 | 2211 | 2.0 | University of Toronto | 10281 | grid.17063.33 | 10281 | Toronto | 392 | Canada | ... | University of Toronto | Ontario | [Education] | NaN | 11 Medical and Health Sciences | 2.0 | 3.81 | 236.5 | 2.0 | 0.0 |
3 | grid.21107.35 | 2211 | 2.0 | Johns Hopkins University | 10120 | grid.38142.3c | 16932 | Cambridge | 845 | United States | ... | Harvard University | Massachusetts | [Education] | NaN | 11 Medical and Health Sciences | 1.0 | 4.99 | 61.5 | 1.0 | -1.0 |
4 | grid.21107.35 | 2211 | 2.0 | Johns Hopkins University | 10120 | grid.17063.33 | 10281 | Toronto | 392 | Canada | ... | University of Toronto | Ontario | [Education] | NaN | 11 Medical and Health Sciences | 2.0 | 3.81 | 236.5 | 3.0 | 1.0 |
... | ... | ... | ... | ... | ... | ... | ... | ... | ... | ... | ... | ... | ... | ... | ... | ... | ... | ... | ... | ... | ... |
3721588 | grid.256592.f | 2219 | 2.5 | Grinnell College | 2 | grid.7359.8 | 3 | Bamberg | 1 | Germany | ... | University of Bamberg | NaN | [Education] | NaN | 19 Studies in Creative Arts and Writing | 130.0 | 33.33 | 8.0 | 8.0 | 5.5 |
3721589 | grid.256592.f | 2219 | 2.5 | Grinnell College | 2 | grid.11560.33 | 2 | Bernal | 1 | Argentina | ... | National University of Quilmes | NaN | [Education] | UNQ | 19 Studies in Creative Arts and Writing | 130.0 | 50.00 | 2.5 | 2.5 | 0.0 |
3721590 | grid.256592.f | 2219 | 2.5 | Grinnell College | 2 | grid.15866.3c | 2 | Prague | 1 | Czechia | ... | Czech University of Life Sciences Prague | NaN | [Education] | CULS | 19 Studies in Creative Arts and Writing | 130.0 | 50.00 | 2.5 | 2.5 | 0.0 |
3721591 | grid.256592.f | 2219 | 2.5 | Grinnell College | 2 | grid.241104.2 | 2 | Cleveland | 1 | United States | ... | University Hospitals of Cleveland | Ohio | [Healthcare] | NaN | 19 Studies in Creative Arts and Writing | 130.0 | 50.00 | 2.5 | 2.5 | 0.0 |
3721592 | grid.256592.f | 2219 | 2.5 | Grinnell College | 2 | grid.256592.f | 2 | Grinnell | 1 | United States | ... | Grinnell College | Iowa | [Education] | NaN | 19 Studies in Creative Arts and Writing | 130.0 | 50.00 | 2.5 | 2.5 | 0.0 |
3721593 rows × 23 columns
Note
The Dimensions Analytics API allows to carry out sophisticated research data analytics tasks like the ones described on this website. Check out also the associated Github repository for examples, the source code of these tutorials and much more.